This is Part II of a two-part post. Part I outlines the process of presenting the data using Tableau and Part II delves into insights from the analysis.
This dashboard was done up for a #VizforSocialGood project.
Discussion:
- The main message I'm trying to bring out is the demand for homes is going up but the supply is going down. And if this trend continues, the problem of homelessness is very likely to perpetuate and there is a need for policymakers to do something to increase the supply of homes. With an increase in supply, this will affect how landlords set their equilibrium, and benefit homeseekers; we can start with places that see a greater average decline over the years.
- Also, another point I wanted to highlight is London district being hit by homelessness worse than other districts in the UK. However, it is not quite easy to predict the change year-on-year as we see great fluctuations over the past years. The only trend we are most likely to be sure is that homelessness is increasing.
- It's interesting how using the same dataset, there are different points of emphasis/ messages that people bring out. Click here to see other visualizations.
Unlike other visualizations, I had chosen not to rank the districts because it could possibly be due to size of problem (in terms of rough sleeper counts) or solution (in terms of vacant dwellings) being correlated with size of districts (potential for further analysis). Hence, I did not want to highlight any district (other than London) in particular before verifying if the challenge was inherent due to natural geographical constraints or a result of differences in local authority management/ landlord expectations. However, this could be overcome by computing a ratio of rough sleeping: vacant dwellings count, which acts as a form of normalization (done in one of the visualizations in the link).
Another main difference is how the other examples try to show that the supply is still sufficient as it is still much more than the number of rough sleepers (eg. 44:1). Hence, seeming to propose that landlords need to step up to the game and reverse the tide (not so much local authorities or policy makers). This positioning is most likely a result of cultural difference; in Singapore, a lot of things are driven top-down rather than bottom-up and ensuring the well-being of citizens is seen as a responsibility of government here.
- This is where we see data science is both a science and an art. Because facts have to be accurately represented, but how we frame the facts leads to a kind of understanding of an issue, as with any art, there is no right or wrong. The variance in stories told by the visualizations will bring about different kinds of calls to action. From the examples in the link above, there are some differences in figures/ top 10 districts presented even though the same datasets are used. It is worth exploring how the data is being transformed/ analyzed/ represented.
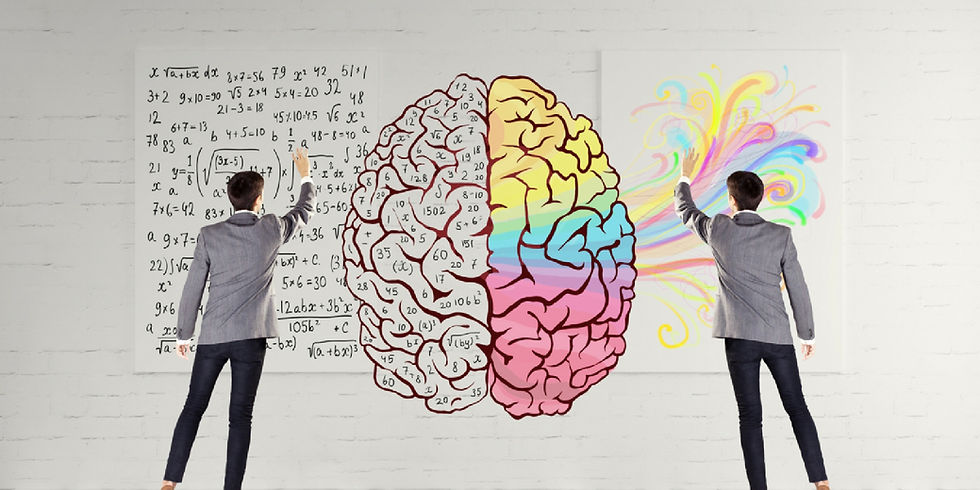
- I also learnt that visualizations that are more sparse on a big dashboard canvas (asking people to scroll more is alright) is more aesthetically appealing. Somehow, I have a tendency to constraint my dashboards to smaller dimensions.
Alternatively, the interactive Tableau dashboard can be viewed here.