Challenges at the start of a data project
- datadoubleconfirm
- Aug 11, 2019
- 2 min read
As with my other posts, I am using the title "data scientist" loosely because titles are not consistently used across the industry so to me, it is a broad umbrella term that covers any type of work that requires one to perform a lot of data analysis or modelling.
There are many types of challenges (and when I say challenges, I meant them as issues/ questions for us to think about/ solve rather than roadblocks/ obstacles) a data scientist might face during his/ her course of work, present within each stage of the CRISP-DM (the CRoss Industry Standard Process for Data Mining) framework. Many also agree that 70% of the time is spent on data cleaning and 30% of the (finally exciting?) work is then spent on modelling. However I would say there are some exciting work at the start of a data project as well if we look at the Business Understanding phase within the 70%; getting to understand how the business work, the problems faced, and the data collected while working with different teams/ business functions is exciting to me.
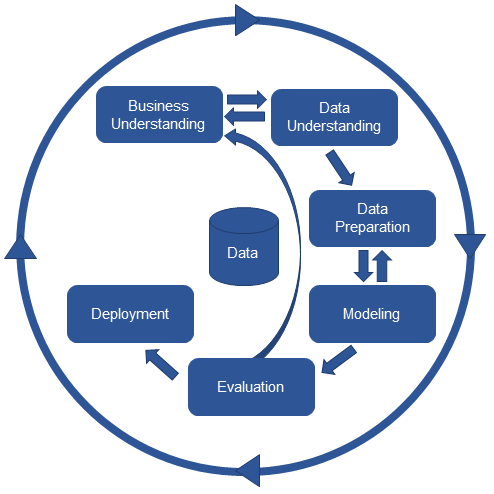
CRISP-DM framework
1. What are the questions business have? Or what is the KPI business is trying to achieve?
2. What data do we have? Where do the data come from? (We want to know if the data is going to be consistently available/ if we can rely on this source to ensure up-to-date information or analysis.) What can we do with the data we have?
3. What data do we need? What kinds of analysis can we then do if we have those data (or else what can we only do?)
During this phase, we can also help think if there's any way to get the data we want more readily as well as innovate on the various methodologies that can be used to analyze the data, through research.
Kommentare