The Typical Data Scientist Profile in 2019 - Featured on request
- datadoubleconfirm
- Feb 7, 2019
- 5 min read
Updated: Jan 22, 2021
Here's sharing a research done by 365 Data Science!
-------
‘Data scientist’ is easily one of the best professions in the world right now. Not only that but it is also one of the newest. This makes things a little tricky for anyone who wishes to enter the field, as discovering which path to take can be like following a dirt trail through a dark forest. All the while, other careers enjoy a well-maintained highway, with road signs, streetlights and GPS.
That’s why it’s important to have a clear picture of what the typical data scientist looks like. In this article, we will draw that picture by using the most reliable method we know – analysing the data.
Last year, we at 365 Data Science performed a study on 1,001 data scientist’s LinkedIn profiles and built what you could call the ‘typical’ data scientist.
Of course, a lot can change in the course of a year, especially in the tech-related industries. That’s why we decided it is important to replicate the study with new data. The purpose of replicating it is to see how things have changed for 2019. Are the most valued skills of a data scientist still the same? How about educational background or country of employment? Well, let’s find out.
Note on data collection: the cohort was divided into two groups depending on whether a person was employed by a Fortune 500 Company (40%) or not (60%). In addition, the sample included data scientists working in the US (40%), UK (30%), India (15%), and other countries (15%). Convenience sampling was employed due to data accessibility limitations.
What is the typical data scientist in 2019?
Great question, because at first glance it doesn’t look like a lot has changed since 2018! It is still a field dominated by men (69%) who speak 2 or more languages (spoken languages, not programming – that would be a much higher average). Eight years is the average working life for the data scientist and 2.3 of those are in the data science field.
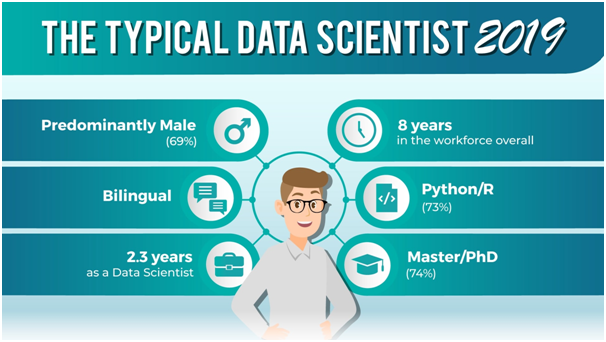
Python and R are still the dominating the programming languages, with 73% of our sample being able to speak at least one of those.
But what does it mean for those who do not fit into this package of ‘typical’ data scientist?
Well as we segment the results further, we get to see a clearer picture.
The education of the data scientist
The first thing we should look at is the education of the data scientist. It’s not surprising to see that 74% of data scientists hold a master’s degree or higher. For a profession with an average salary of $117,000, this is understandable.
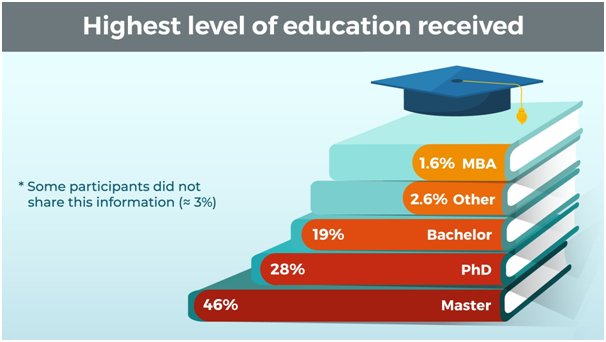
However, while some may expect PhD holders to make up the brunt of data scientists, they would be wrong, as only 28% hold PhDs. Compare this to the 24.2% who have a bachelor’s or below, and we have surprisingly wide range of educational backgrounds.
But if any aspiring data scientists were to invest the time and effort, it seems a master’s is still the most sensible route with 46% of the sample boasting this particular level of academic prowess.
That said, it is worth nothing that the number of data scientists with bachelor’s degrees has increased by 4% since last year, so we could be seeing a trend where more data scientists with a lower level of education enter the field.
In addition, there has been a 3% increase in the number of data scientists who have taken the initiative and gained online certifications (43% compared to last year’s 40%). Therefore, we may have a trend developing here as well.
What are the benefits of self-preparation and online courses?
The study does tell us that almost all data scientists have some level of formal education, however, the areas of studies that data scientists pursue are sporadic because there is not a particularly strong infrastructure in formal data science education. Only 12% of our cohort’s academic studies were actually in data science or data analysis.

In fact, the data shows that a vast number of data scientists come from other academic backgrounds and tailor their skill set through self-preparation and online courses (43%); and with the average number of certificates being 3, it seems like a customized set of talents is the way forward.
It’s also worth noting that many LinkedIn profiles do not show all certificates, as many of us do not post irrelevant things. For example, one would not boast a certificate in “The Fundamentals of Python” when they have been a senior python programmer for 3 years. That said, we expect the percentage of certificate holders to be much higher than 43%.
This is good news for those of you who do not have the time or resources to complete a master’s or a PhD but could easily manage a few online courses in between your already hectic schedules.
Without a solid curriculum, what skills make the most sense to acquire?
As was mentioned earlier, you’ll be hard pressed to find a data scientist who doesn’t speak at least one programming language. Therefore, it’s not surprising that it is coding skills that dominate a data scientist’s professional toolbox, and which seem to be more valued than knowledge of software like Tableau or Excel.

In the last few years Python and R have been battling for title of ‘most used language in the data science field’, however it is Python that is making headway, leaving R in second place with a solid 10% between the two, worldwide. It is interesting to see, however, that the UK favors Python more than R (UK data scientists who speak Python are 57%, compared to R-speakers who are 39% of the cohort). In both the US and India, the two languages seem to be equally valued. In the remaining countries, however, the trend appears again, with Python being preferred over R by and large (63% compared to 40%). It will be curious to see how this develops in the years to come.
SQL sits comfortably in 3rd place as relational database usage seems consistent across the globe, while Matlab and Java are trailing the pack with only 19% and 18% of data scientists boasting the skill, respectively. It appears they’re losing their relevance and we could see their numbers drop even further as Python’s and R’s solidly rise.
Conclusion
We can already see that while there are trends to the attributes of a data scientists, it is in no way clear cut. Of course, there are more factors in play when it comes to determining a ‘typical’ data scientist, such as university rank, previous employment and even current company size. We delve into these areas in the full study which can be found here, so if your curiosity has peaked, go check it out.
Overall, however, the study shows that compared to other well-paid professions the path to becoming a data scientist is much more flexible.
That said, the goal here was not to dissuade aspiring data scientists but to inspire them to go out and achieve their goals. With a good amount of information, motivation, and determination, anyone can make it in one of the most exciting careers of the 21st century.
Here's the full infographic.

------
If you're interested in what the various tools are used for (and why do we need so many of them), check out this post I had previously written! And also, I get the question on how to get started quite a lot, so I wrote a post on this as well :)
Kommentit